Collaborating With Computers To Improve Cancer Treatment
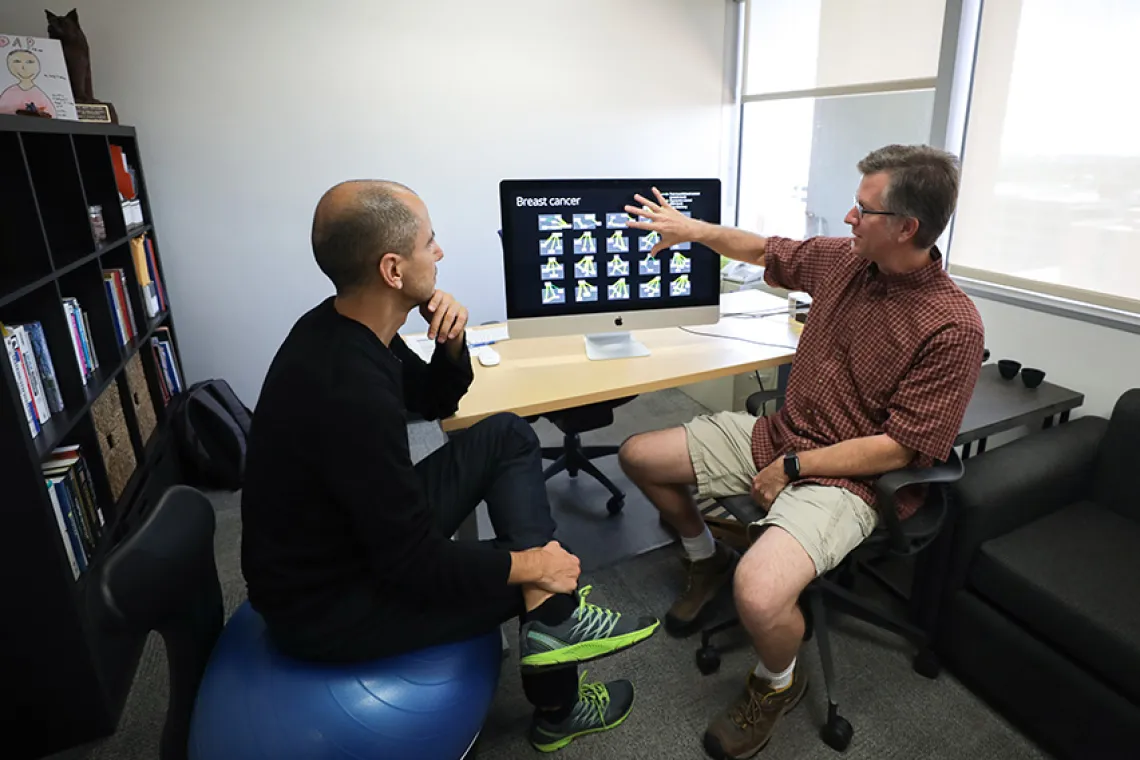
Clayton Morrison (right) with Mihai Surdeanu.
Photo by Anna Augustowska.
More than a million research papers on cancer are published every year, detailing results that inch us closer to understanding cancer pathways, the molecular interactions that cause cells to become cancerous. Each finding only contains a small piece of the puzzle. But taken together, analyzed as a collective body of data, this research can lead us to fast, effective, and more individualized, medical care.
There is no way a single person – even someone with the most advanced expertise – can read and synthesize such enormous amounts of data. That is where University of Arizona researchers come in.
Clayton Morrison, an associate professor in the UA School of Information in the College of Social and Behavioral Sciences, is a co-principal investigator of a $3.6 million research grant funded by the Defense Advanced Research Projects Agency, or DARPA. The project, titled "REACH: Reading and Assembling Contextual and Holistic Mechanisms From Text,” is part of DARPA’s Big Mechanism Program, which aims to develop automated technologies for a new kind of science in which research is immediately integrated into causal, explanatory models.
The UA REACH team – led by principal investigator Mihai Surdeanu, associate professor in the Department of Computer Science – includes Ryan Gutenkunst and Guang Yao from the Department of Molecular and Cellular Biology; Kobus Barnard, a professor of computer science; and several graduate students from linguistics, information and computer science. They are joined by Emek Demir, a computational biologist at Oregon Health & Science University.
“This is a problem whose solution requires both biological and computation and information scientists working together,” Morrison said.
Morrison contributed to the machine-learning component of the project, teaching the computer how to identify the context of biochemical interactions and how to determine when a paper is relevant to answering a specific question. He also helped develop a statistical framework for composing networks of interactions that form cancer pathways.
The team ultimately developed a natural language processing system that can read biomedical research papers and extract the protein signaling pathways discussed with a precision approaching that of a human cancer researcher. The big difference: The REACH program can parse an entire paper in 10 seconds.
The data is then plugged into large-scale, interactive models. This work is more than just collecting mountains of data. The researchers are building algorithms to synthesize and draw connections, training the computers to make inferences from the data.
“REACH has the potential to discover new, previously missed, cancer-driving mechanisms by aggregating fragments of information from different publications,” Surdanu said. “We demonstrated that REACH helps identify and explain novel cancer-driving mechanisms for seven different cancers.”
REACH researchers are laying the foundation for interactive software that would allow doctors to enter patient health and genome data. The causal models could then predict how an individual’s specific cancer might respond to treatment.
Morrison said he hopes the work will have a direct impact on creating effective cancer drugs and personalized medical treatment.
“I am excited because this work can have a direct impact on helping us better understand cancer,” Morrison said. “This work is pushing toward the dream of developing computer systems that become real collaborators in helping us better understand and solve very complex problems.”